Oliver Limoyo
Ph.D. StudentDepartment: Current Students, PhD Students
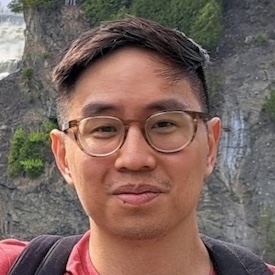
Self-supervised learning and generative modelling are promising paradigms for data-driven robotics as they do not require explicit labels. Oliver is interested in exploring how these approaches to learning can be applied to or used to re-interpret classical robotic problems such as system identification, inverse kinematics and pick-and-place.
Oliver Limoyo is a PhD candidate in the STARS Laboratory. During his studies, he completed a year-and-a-half-long internship at Samsung AI Center in Montreal as an Applied Reinforcement Learning Researcher and a four month internship at Kindred AI. He previously completed a B. Eng in Mecahnical Engineering at McGill University in Montreal, Quebec, Canada.
Generative Modelling for State Space Models
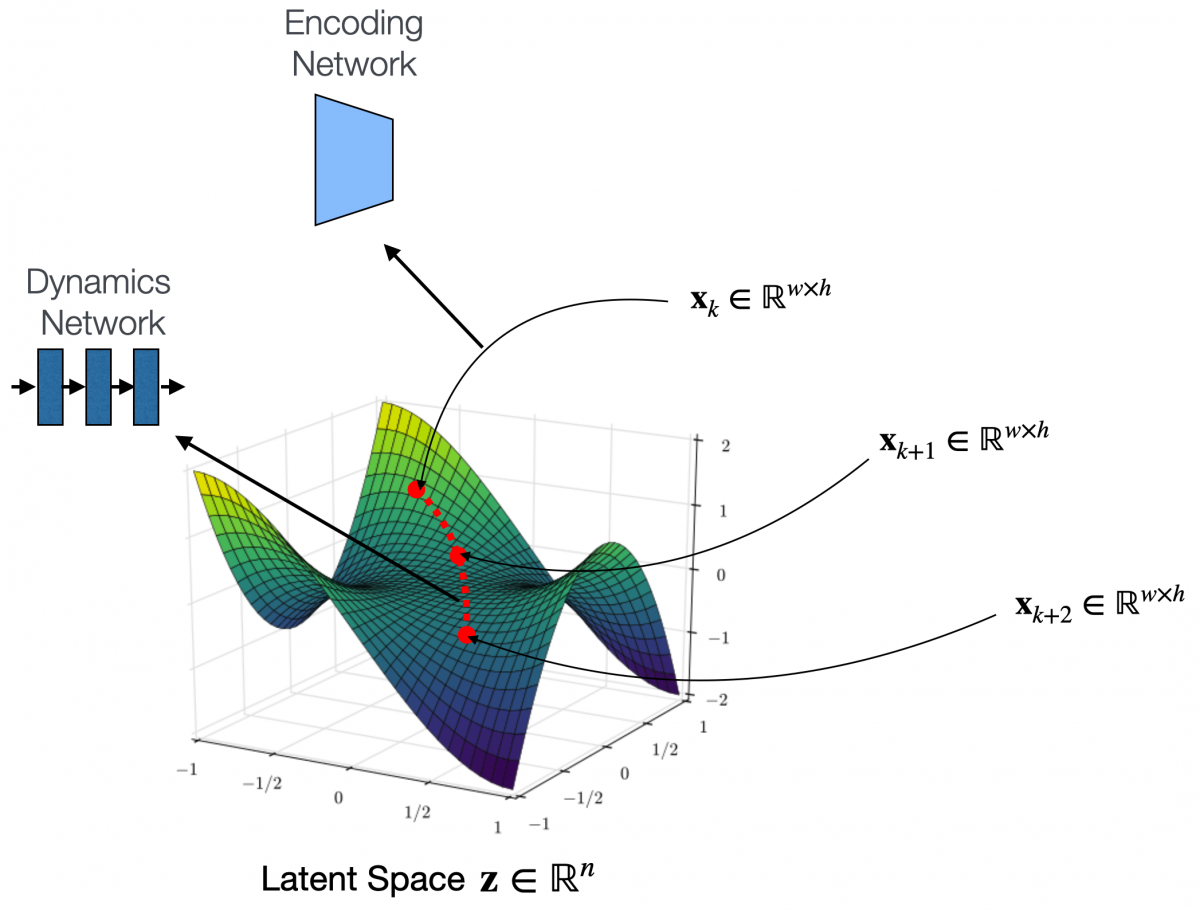
We embed high-dimensional observations into a lower-dimensional latent space in which a state space model can be learned.
Oliver Limoyo, Bryan Chan, Filip Marić, Brandon Wagstaff, Rupam Mahmood and Jonathan Kelly
RA-L (2020)
Learning Sequential Latent Variable Models from Multimodal Time Series Data
Oliver Limoyo, Trevor Ablett and Jonathan Kelly
IAS (2023).
Self-Supervised Learning for Pick-and-Place
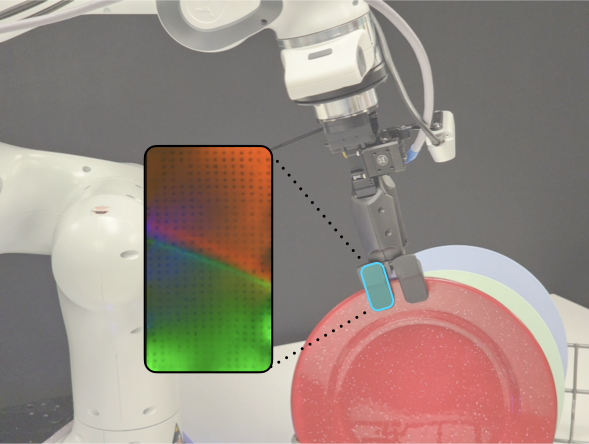
We approach the learning of robotic object placement policies by reversing the grasping process and exploiting the inherent symmetry of the pick and place problems. Our system is capable of collecting hundreds of demonstrations without human intervention by using a combination of tactile sensing and compliant control for grasps.
Oliver Limoyo, Abhisek Konar, Trevor Ablett, Jonathan Kelly, Francois Hogan and Gregory Dudek
Submitted to ICRA’24
Generative Modelling for Inverse Kinematics
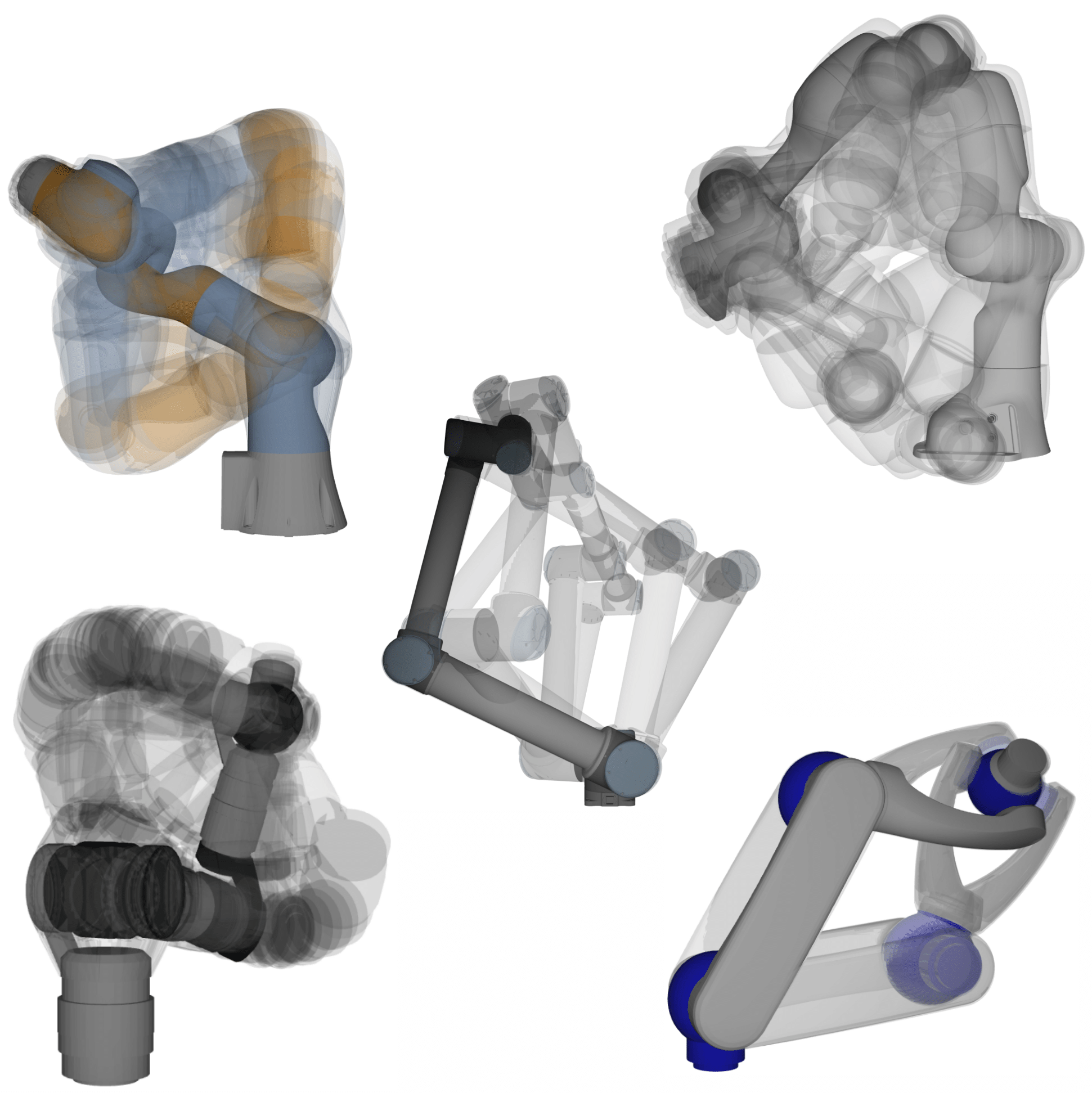
Our generative graphical inverse kinematics (GGIK) model is capable of simultaneously representing general (i.e., not tied to a single robot manipulator model or geometry) IK mappings and to produce approximations of entire feasible sets of solutions.
Oliver Limoyo*, Filip Marić*, Matthew Giamou, Petra Alexson, Ivan Petrović and Jonathan Kelly
Submitted to T-RO (2023).
Oliver Limoyo*, Filip Marić*, Matthew Giamou, Petra Alexson, Ivan Petrović and Jonathan Kelly
Workshop on Symmetries in Robot Learning RSS (2023).
Self-Calibration of Mobile Manipulator Kinematic and Sensor Extrinsic Parameters Through Contact-Based Interaction
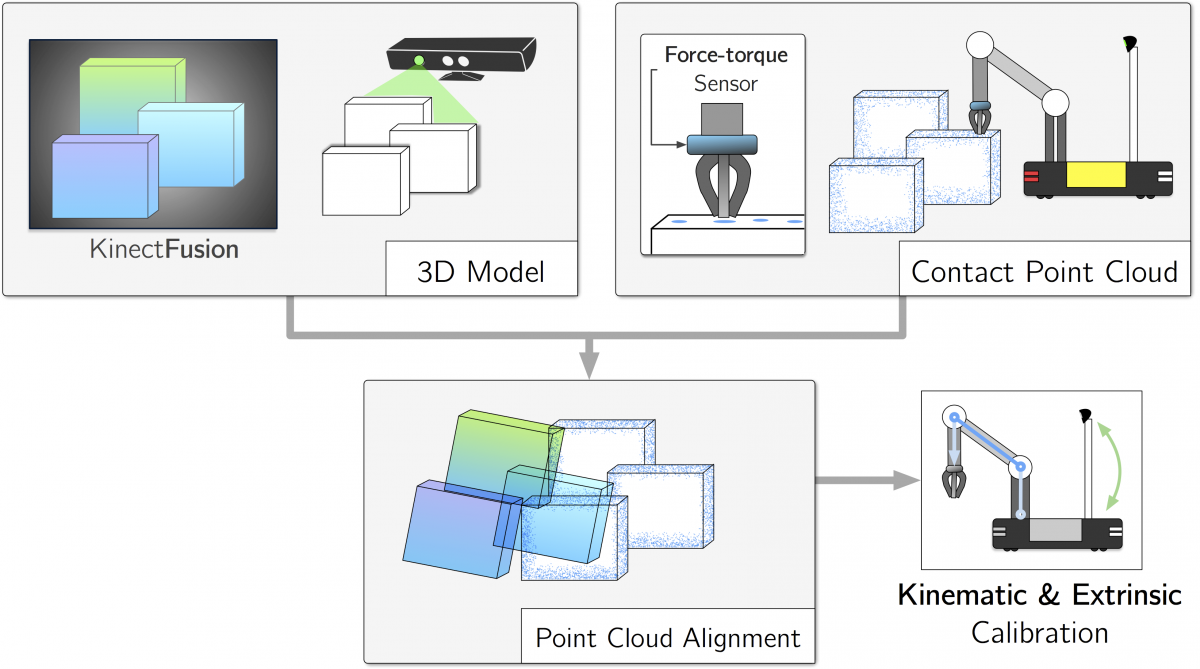
Our contact-based self-calibration procedure exclusively uses its immediate environment and on-board sensors.
Oliver Limoyo, Trevor Ablett, Filip Marić, Luke Volpatti and Jonathan Kelly
ICRA (2018).