Dr. Filip Marić
Ph.D. Student (2023)Department: Alumni
Motion planning is one of key challenges in robotics today. When determining how a robot should perform a task, environmental and safety factors should be considered. For example, obstacles in the environment should be avoided, while also taking into account sensor measurement uncertainty and possible energy restrictions. Currently, there are many different ways of approaching this problem, ranging from classical optimization to deep learning. Filip developed motion planning algorithms with a focus on manipulators. He worked as a member of STARS and with the LAMoR group at the University of Zagreb.
Manipulability Optimization for Manipulator Motion
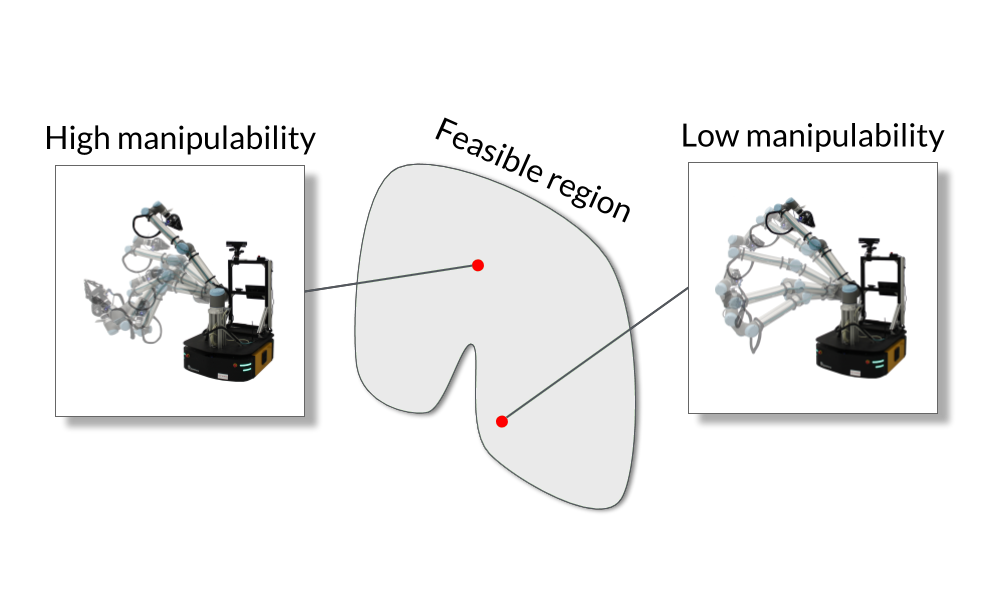
High and low manipulability variants of trajectories for performing the same task.
Filip Marić, Luka Petrović, Marko Guberina, Jonathan Kelly, Ivan Petrović
Robotics and Autonomous Systems (2021)
Filip Marić, Oliver Limoyo, Luka Petrović, Trevor Ablett, Ivan Petrović, Jonathan Kelly
IROS (2019)
Global Polynomial Optimization for Robot Kinematics
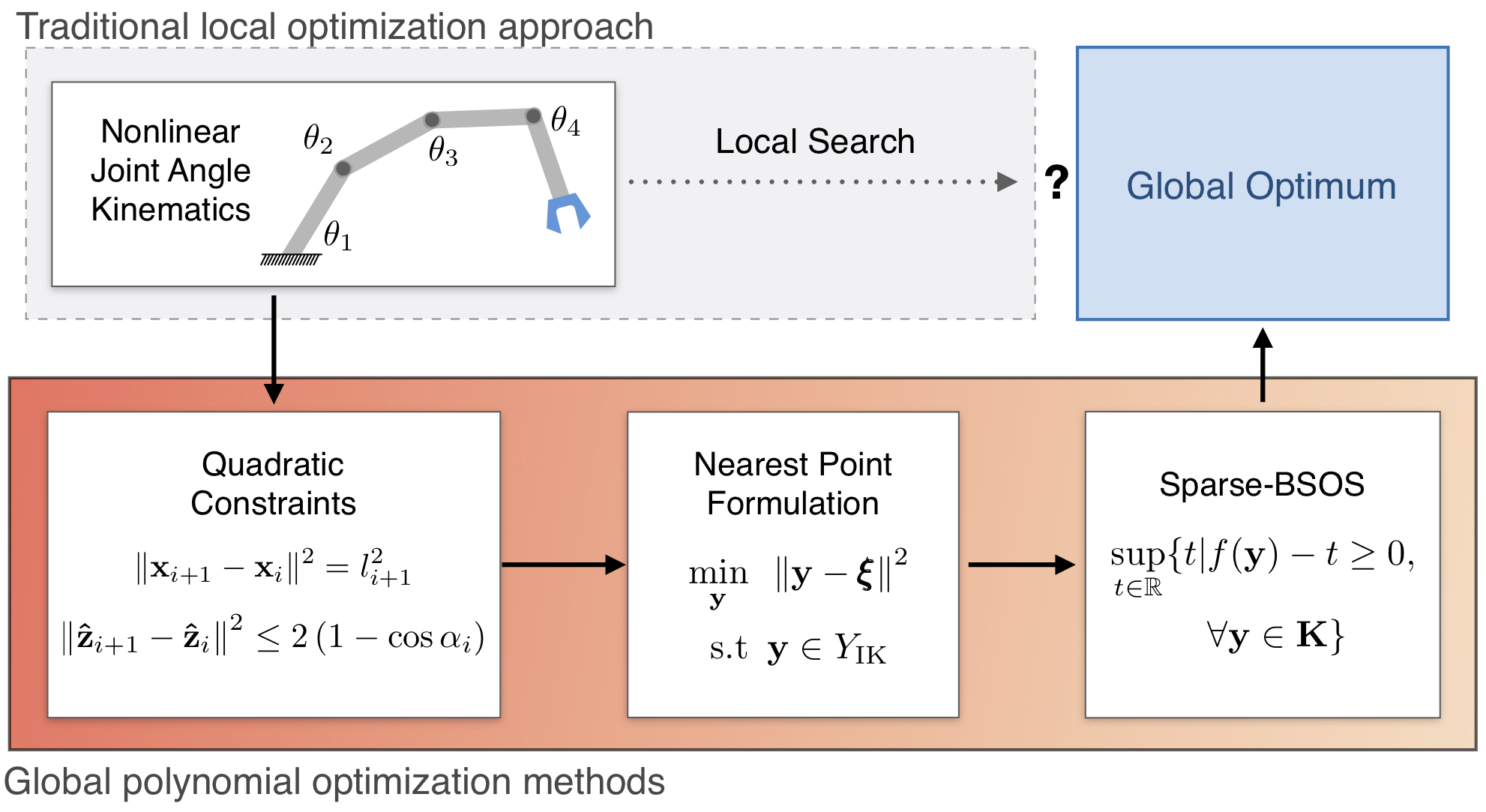
Convex relaxations for polynomial formulations of inverse kinematics.
Matthew Giamou*, Filip Marić*, David M. Rosen, Valentin Peretroukhin Nicholas Roy, Ivan Petrović, Jonathan Kelly, ICRA (2022)
Filip Marić, Matthew Giamou, Soroush Khoubyarian, Ivan Petrović, Jonathan Kelly
ICRA (2020)
Distance-Geometric Inverse Kinematics
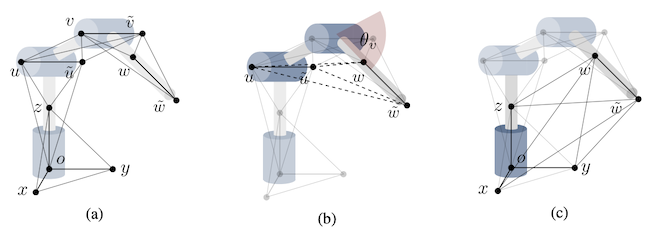
Encoding the configuration of a manipulator as a partially complete graph.
Filip Marić, Matthew Giamou, Adam W. Hall, Soroush Khoubyarian, Ivan Petrović, Jonathan Kelly
IEEE TRO (2022)