Dr. Brandon Wagstaff
Doctoral Candidate (2022)Department: Alumni
Brandon’s research focussed on using low-cost sensors (cameras and IMUs) for navigation and localization. In particular, he investigated how deep neural networks could be combined with classical estimators to yield better overall performance, under nominal and degraded conditions. Ultimately, his work is intended to produce algorithms that are able to operate within challenging environments, where classical algorithms are prone to failure.
For example, classical algorithms commonly rely on parameter tuning/calibration, which is highly sensitive to an agent’s motion or to the environment that the agent operates within. One of Brandon’s goals was to obviate the need for calibration or parameter tuning by replacing the sensitive components of the system with more robust learning-based models. By doing so, these systems are better able to operate within continuously-changing environments and over longer periods of time. He worked on several projects including:
Foot-Mounted Inertial Navigation for Indoor Localization
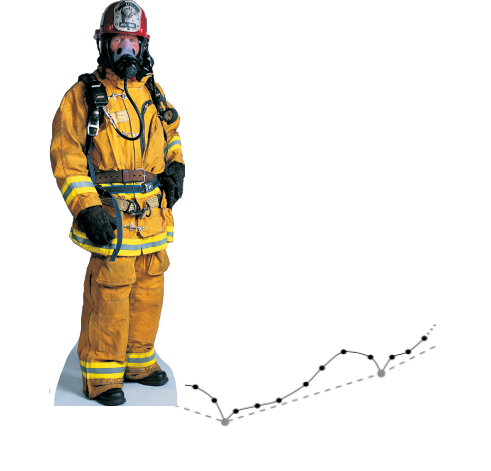
Foot-mounted inertial sensing can be used for first-responder localization.
Brandon Wagstaff, Valentin Peretroukhin, and Jonathan Kelly, IPIN (2017)
LSTM-Based Zero-Velocity Detection for Robust Inertial Navigation
Brandon Wagstaff and Jonathan Kelly, IPIN (2018)
Robust Data-Driven Zero-Velocity Detection for Foot-Mounted Inertial Navigation
Brandon Wagstaff and Jonathan Kelly, IEEE Sensors J. (2020)
Deep Measurement Models for Visual-Inertial Navigation
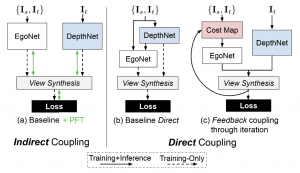
The type of coupling between depth and egomotion networks has a significant effect on navigation performance.
Brandon Wagstaff, Valentin Peretroukhin, and Jonathan Kelly, ICRA (2020)
Self-Supervised Scale Recovery for Monocular Depth and Egomotion Estimation
Brandon Wagstaff and Jonathan Kelly, IROS (2021)
On the Coupling of Depth and Egomotion Networks for Self-Supervised Structure from Motion
Brandon Wagstaff, Valentin Peretroukhin, and Jonathan Kelly, IEEE RA-L (2022)
A Self-Supervised, Differentiable Kalman Filter for Uncertainty-Aware Visual-Inertial Odometry
Brandon Wagstaff, Emmett Wise, and Jonathan Kelly, IEEE AIM (2022)